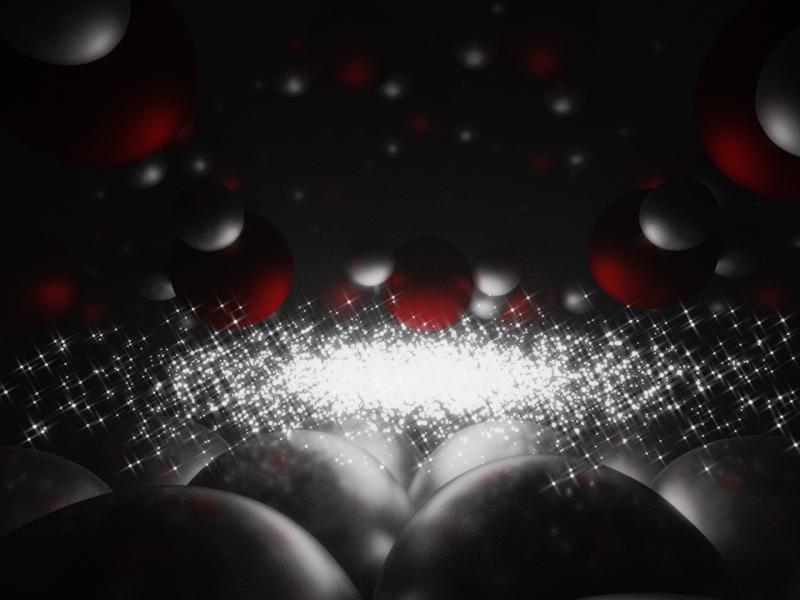
Accurate predictive simulations of the electrochemical reactions that power solar fuel generators, fuel cells, and batteries could advance these technologies through improved material design, and by preventing detrimental electrochemical processes, such as corrosion. However, electrochemical reactions are so complex that current computational tools can only model a fraction of all relevant factors at one time — with limited accuracy. This leaves researchers reliant on the trial and error of significant and expensive experimentation.
With the support of a $2.6 million grant from the Department of Energy, Ravishankar Sundararaman, an associate professor of materials science and engineering at Rensselaer Polytechnic Institute, in collaboration with a multi-institution team of researchers, is taking on the challenge of developing accurate, cost-effective, and highly accessible computational electrochemistry tools.
“Our project aims to address the full complexity of electrochemical systems by combining quantum electronic simulations with classical electrolyte models,” said Sundararaman. “Then, by using AI to learn from all previous simulations, we also hope to predict electrochemical processes quickly and accurately.”
Researchers currently use a quantum electronic simulation method known as density functional theory (DFT) to predict electrochemical reactions. While DFT is an accessible and affordable tool, Sundararaman said, it’s not always accurate enough to simulate complex electronic interactions, limiting the quality of research predictions.
The Rensselaer team will work with researchers at the National Renewable Energy Laboratory, Lawrence Berkeley National Laboratory, the University of Colorado at Boulder, and the University of South Carolina to develop improved simulation tools, beyond the abilities of DFT that also account for electrolyte solvation that happens at electrode surfaces.
Researchers will use supercomputers like AiMOS at Rensselaer to apply their new modeling techniques on several electrochemical systems, creating an open database of these simulations. They will then use machine learning and AI tools, combined with the new database, to enable accurate predictions for new materials without performing expensive simulations. This way, even without access to a supercomputer, the broader research community will be able benefit from their work.
“Our database will capture how standard DFT and our new beyond-DFT techniques for electrochemistry differ,” Sundararaman said. “We essentially hope to use all these computing resources to then provide that beyond-DFT level of accuracy for electrochemical materials design at a fraction of the cost.”