We address the problem of learning of classical Markov Random Fields that are widely used in material science, statistical physics, and computer science to represent structured Gibbs distributions. We introduce a new computationally efficient Interaction Screening method for learning discrete and continuous Gibbs distributions for which maximum likelihood approaches are intractable. The algorithm recovers the structure and parameters of the Hamiltonians with multi-body interactions specified in an arbitrary basis. A rigorous sample complexity analysis of the Interaction Screening estimator shows systematic improvement in the number of required experimental or simulation samples compared to all previously known bounds.
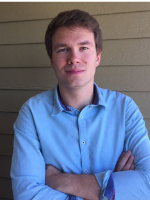
Dr. Andrey Lokhov received his M.Sc. degree in theoretical physics from Ecole Polytechnique and Ecole Normale Superieure, France, and a Ph.D. degree in statistical physics from Universite Paris-Sud, France. He is currently a Staff Scientist in the Theoretical Division at Los Alamos National Laboratory. His recent research interests include theory and algorithms in statistical learning, inference and optimization problems on networks, and quantum computing.