High-fidelity physics-based simulations have been widely developed to study complex physical systems. Despite its interpretability and accuracy, physics-based simulations usually have two limitations: a) running high-fidelity simulations often requires high computational cost and consumes a large amount of time; b) most of the simulations are built upon deterministic methods that is insufficient for uncertainty quantification (UQ) when modeling complex systems with various types of uncertainties. This presentation will introduce two examples of building engineering-driven surrogates for high-fidelity simulations to significantly improve its computational efficiency while preserving its accuracy. (1) A spatial-temporal data fusion method, StressNet, is proposed to predict the maximum internal stress within the material along with the fracture propagation. The great fluctuation in the maximum internal stress is accurately captured by fusing the fracture propagation into the prediction; (2) Neural process aided ordinary differential equation (NP-ODE) is proposed to bridge the gap between the physics-based simulation method and the data-driven surrogate. NP-ODE is proposed by incorporating the idea of modeling and solving differential equations into designing the stochastic process and demonstrates outstanding performance in recovering the underlying pattern from the noisy dataset and conducting uncertainty quantification.
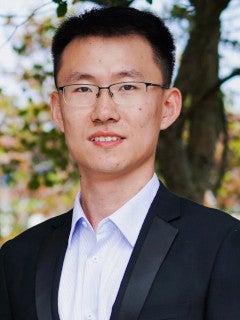
Dr. Yinan Wang is a tenure-track Assistant Professor in the Department of Industrial and Systems Engineering at Rensselaer Polytechnic Institute (RPI). Before joining RPI, he received the Ph.D. degree in Industrial and Systems Engineering from Virginia Tech in 2022, the M.S. degree in Electrical Engineering from Columbia University in 2019, and the B.S. degree in Electrical Engineering and Automation from Xi'an Jiaotong University in 2017. His research interests include complex data analytics, system pattern recognition, and machine/deep learning techniques in complex physical systems. His research is recognized as the Best Ph.D. Dissertation Award from Computer & Information in Engineering (CIE) division at ASME, seven best paper awards/finalists (e.g., IISE Transactions Best Paper Award, etc.), and one featured article in ISE magazine. He is a recipient of the FTC Early Career Grant Award from the American Society of Quality and is selected as the Panel Fellow in the NSF CMMI Game Changer Academy.