Traditionally materials design relies on empirical methods such as trial-and-error, high-throughput screening, or combinatory experimentation. In contrast, inverse materials design adopts a systematic and computational approach to identify and engineer materials suitable for specific purposes. In this talk, I will present case studies highlighting the power of molecular theory and machine learning for the inverse design of nanostructured materials for chemical separation, wet adhesion, and electrochemical energy storage.
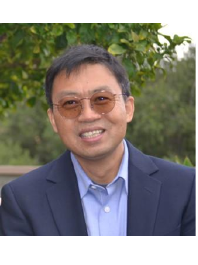
Professor Wu is a faculty member in the Department of Chemical and Environmental Engineering at the University of California, Riverside. He holds a PhD from the University of California, Berkeley, along with MS and BS degrees from Tsinghua University, Beijing. He is an elected Fellow of the American Physical Society (APS) and of the American Institute for Medical and Biological Engineering (AIMBE).