Innovative materials are needed to tackle current major challenges in energy storage and generation. However, the design of new materials largely relies on experimental trial and error, limiting the number explored compounds relative to the entire space of possible compounds. In this presentation, I will discuss our approach to materials design, which integrates machine learning (ML) techniques with quantum mechanics-based computations. Our ML models are trained to predict quantum mechanical properties, like formation energy and band gap, using graph neural network that input cheap proxy structures as input. I'll highlight a few applications in computational materials design where ML offers significant advantages, such as circumventing costly electronic structure calculations to provide statistical approximations of high-fidelity band structures. This allows for an improved computational understanding of a material's electronic properties.
One limitation of using structure-based ML models in material screening is due to the prerequisite of having a well-defined structure for optimal accuracy. To address these challenges, we have developed a generalizable ML-based interatomic potential (MLIPs) for structure prediction of complicated organic/inorganic materials. Additionally, these MLIPs are highly computationally efficient which allows for modeling the temperature-dependent dynamics of local structural domains. Such insights can be valuable when assessing a material's suitability for energy-based applications. Overall, the application of ML and computation shows great promise in facilitating the exploration of the chemical space to allow for transformative advancements in energy storage and generation.
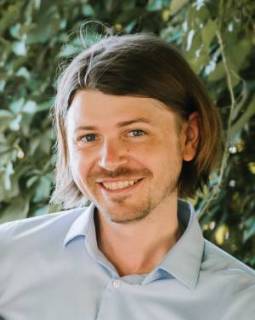
Dr. Sutton finished his PhD from Georgia Tech under the supervision of Jean-Luc Bredas. After a 6-month postdoc with Weitao Yang at Duke University, he joined the Fritz-Haber Institute in Berlin as an Alexander von Humboldt postdoctoral fellow from 2015 to 2020. Chris then joined the Chemistry/Biochemistry Department at the University of South Carolina in 2021.